This is the multi-page printable view of this section. Click here to print.
Articles
- Understanding the Operational Costs of Rusty CloudWatch Exporter
- A Better AWS CloudWatch Exporter: Ingestion Architecture Overview
- The Unsatisfying State of Prometheus-Based Monitoring of AWS Infrastructure
Understanding the Operational Costs of Rusty CloudWatch Exporter
Originally posted in LinkedIn
Understanding the impact of deploying monitoring tools on operational costs is essential for making informed decisions in cloud infrastructure management. In this blog post, we delve into the specifics of Rusty CloudWatch Exporter’s design and explore how it influences operational costs. By arming potential users with this knowledge, we aim to facilitate a deeper understanding of whether this tool aligns with their budgetary constraints and operational requirements.
Outside of the scope of this post is to analyze the infrastructure used to host the exporter as there are so many variations with completely different profiles that wouldn’t be feasible to analyze. Thus, we are going to focus on the costs of using the streaming architecture to get the metrics out from AWS CloudWatch into the exporter. Let’s dive in…
The starting place to do the analysis is from the overview of how Rusty CloudWatch Exporter fits into the AWS ecosystem to do the metric translation:
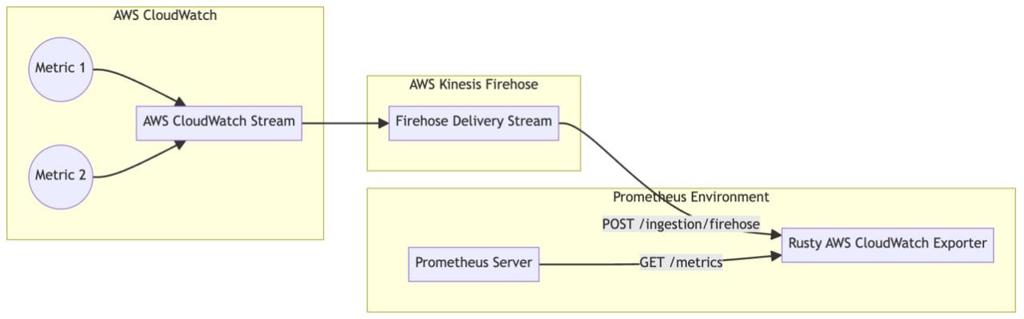
As the exporter uses the CloudWatch Metric Stream mechanism along with an HTTP Endpoint, the operational costs will primarily come from three items: Metric Stream costs, Firehose Ingestion costs and Firehose Outbound Data Transfer costs.
TL;DR
1-minute frequency metrics will cost approximately $0.13176 per month with lower frequency ones costing proportionally.CloudWatch Metric Streams pricing[1] is really straight-forward: there’s a fixed charge per metric update - in most regions this is $0.003 per 1,000 metric updates.
A metric update will contain enough data to calculate sum, min, max, average and sample count. If other statistics such as percentiles want to be added to a metric it will add an extra metric update for each extra 5 statistics.
How often a metric will get an update depends on the metric itself and, sometimes, on how the services emitting metrics are configured.
The information can usually be found in each of the service’s metrics documentation page. For example, for DynamoDB only 14 metrics are updated at the minute frequency while most of them are updated in 5-minute intervals[4].
And to make things a bit more complicated some metrics won’t trigger an update unless a specific condition happens: the HTTPCode_Target_5XX_Count
will only emit an update if a target generated a 5XX response in the 60-second period.
The two other charges are based on the amount of data that the Firehose delivery stream has to handle and this is strictly correlated to the number of metric updates that are being shipped. The size of each update will vary based on the number and length of tags and other elements within the structure, but based on own and others observations, a 1KB per update is a fair estimate.
The Firehose Ingestion Costs are straight-forward, there is a flat-fee per GB ingested that will depend on the region[2]; for Oregon it is $0.029/GB. Using the aforementioned estimate, we get that the cost is going to be around $0.029 per 1M metric updates.
The Firehose Outbound Data Transfer costs are contingent upon the deployment location of the Rusty AWS CloudWatch Exporter and its accessibility from Firehose. If the exporter operates outside of AWS, outbound internet traffic costs are incurred. If it operates in a different region from Firehose, inter-region costs apply, whereas intra-region costs are applicable if they are in the same region. These costs[3] typically amount to $0.09/GB, $0.02/GB, and $0.01/GB, respectively, in the most common regions. Additionally, if the exporter is accessed through a VPC, an extra fixed-priced fee of $0.01/hr or approximately $7/month, alongside the $0.01/GB, is levied. Assuming no compression, these figures can be extrapolated to per 1M metric updates.
Adding up all the different items we get that the overall cost per 1M metric updates will be between $3.03 and $3.119. As noted, the actual cost will change based on other factors but, as the bulk of the costs come from the metric streams, the variation will not be substantial. From here to the cost per metric is a simple math calculation: 1-minute frequency metrics will have 43,200 updates per month while 5-minute ones will have only 8,640 updates per month yielding a cost of ~$0.13176 and ~$0.02635 respectively.
How Does It Compare to YACE?
Given that YACE is widely regarded as the default exporter for AWS CloudWatch metrics, it’s pertinent to compare its operating costs with those of our exporter. The primary distinction between them lies in their methods of retrieving metrics from AWS: one utilizes Metric Streams, while the other leverages the CloudWatch API.
The way that YACE works is by, first, doing a ListMetrics for each the different metric names it has been configured to retrieve and then issuing either a GetMetrics
or GetMetricStatistics
for each of the metric instances found in the list call.
It is important to notice that the ListMetrics
will return all instances that have generated data recently but not necessarily for the period that is being scraped.
What this means is that if a metric hasn’t been updated it will still be listed and scraped yielding a NaN
value.
The API call pricing is $0.01 per 1,000 requests for the ListMetrics
and each API call can return up to 500 metrics.
Independently of which API is used to retrieve the metric values, the cost is the same: $0.01 per 1,000 metrics with up to 5 aggregations or stats in each.
In the case of a 1-minute frequency metric, this translates to $0.43286/month.
$0.432 coming from issuing 43,200 GetMetric API calls and $0.00086 from its share of the ListMetrics
calls.
If we are using a 5-minute frequency scraping, the cost will be a fifth of that or $0.08657 per metric per month.
In either case, the operating costs for retrieving the metrics is significantly lower when using the streaming architecture versus the API mechanism. Furthermore, YACE will scrape all metrics at the same interval independently of how frequently they are updated, potentially making 5-minute metrics scraping costs as high as 1-minute frequency metrics.
Where YACE has the upper hand, though, is that it allows reducing the update frequency of the metrics. That is, it can be configured to scrape 1-minute update frequency metrics every 10 mins thus reducing its scraping cost; something not possible when using CloudWatch Streams.
Wrap Up
By using the streaming architecture, we are able to reduce the costs of getting the metrics out of AWS in order to do the translation into a Prometheus compatible format while improving the ingestion delay.
Additionally, through this analysis, we provide an estimation of the anticipated operational costs associated with running the exporter. This empowers you to make informed decisions regarding the feasibility of integrating the exporter into your budget, mitigating the risk of unexpected expenses down the line.
A Better AWS CloudWatch Exporter: Ingestion Architecture Overview
Originally posted in LinkedIn
In my previous post I discussed what I found was lacking in the current tool ecosystem to be able to successfully monitor AWS services.
This will be the first of several posts where I will start exploring and explaining different facets of a tool I’m developing to help fill in those gaps. In this one, I will be focusing on the ingestion mechanism.
The Pain Points
As we’ve seen, the two main tools that exist today rely on AWS CloudWatch API to retrieve the metrics. Because of this polling mechanism, they both require the customer to configure the refresh interval as well as the lookback period to use in each retrieval. The way it works leads to several non-optimal behaviors. Setting a short refresh interval can lead to API throttling or the full set of metrics cannot be retrieve within that interval. A large interval reduces the freshness of the data and can lead to reaction lags when monitoring based on those.
Also, because AWS doesn’t expose the metrics gathered immediately through the CloudWatch API, it can lead to the polling not being able to retrieve values for recent periods leading to gaps in the metrics time-series.
Managing all those knobs is quite painful and balancing all the configurations so that the metrics are retrieved consistently, correctly and with the least amount of lag possible is not easy. It also needs frequent re-evaluation as the infrastructure size changes as well as CloudWatch behavior changes.
Improved Ingestion Architecture: Leveraging CloudWatch Metrics Streams
Fortunately, AWS provides a push mechanism to get AWS CloudWatch metrics as they are generated. These are CloudWatch Metrics Streams. With a CloudWatch stream we can have AWS deliver the metrics as they are generated to a Firehose which itself will deliver them to an HTTP endpoint for them to be processed.
The ingestion architecture for the exporter looks like this:
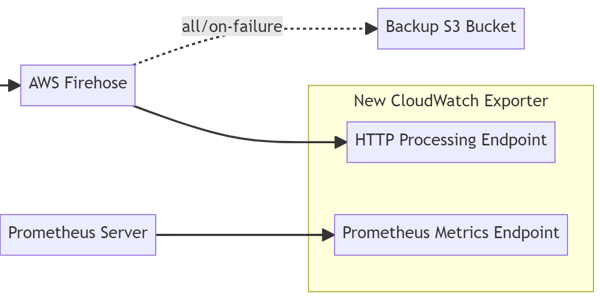
Diagram of The Ingestion Architecture Using CloudWatch Streams
The CloudWatch Stream acts as the generator of metrics while the AWS Firehose acts as the batching and dispatching mechanism to the Rusty AWS CloudWatch Exporter’s HTTP endpoint.
Pros and Cons of Using CloudWatch Streams
CloudWatch Streams were introduced in 2021[1] and it is the preferred mechanism to continuously retrieve metrics generated by AWS CloudWatch. They provide near real-time-delivery and a very low latency, specially compared to the polling mechanism.
By leveraging CloudWatch Streams the exporter gets several advantages over the traditional polling mechanisms:
- Low latency from metric update to metric processing and making it available for Prometheus to scrape it. Lag will be introduced by Firehose batching, but that can be capped at 60 seconds and depending on the volume it can actually be less than that.
- No unnecessary polls for stale or not-generated metrics. The polling mechanism is forced to attempt to retrieve metrics that might either not have changed or not generated at all. Not all CloudWatch metrics are generated by default, some are only generated when certain events happen, for these metrics the Stream will not issue updates unless there are.
- Generated metrics are configured at the source. In the existing solutions one needs to configure the exporter with the metrics one wants to export and some metric discovery criteria. CloudWatch streams has that configuration now and as new metrics are generated that match the configuration, they will be forwarded to the exporter through Firehose.
- It removes the risks of hitting the CloudWatch API throttling limit. It is quite common for large infrastructure footprints to hit this when using the existing exporters, thus requiring to fine tune the concurrency and the update interval to stay within limits.
- It standardizes the ingestion format for the new exporter. Firehose HTTP request format is fairly standard but the important piece is that the CloudWatch metrics are exported in the OpenTelemetry 0.7.0 standard format.
The cons of using CloudWatch Stream is that it requires more setup work:
- Hosting the Rusty AWS CloudWatch Exporter where Firehose can reach it and enable HTTPS for the endpoint.
- Compared to the current exporters which can be run anywhere with access to the AWS CloudWatch API, this setup requires setting up several pieces of infrastructure with appropriate amazon policies: CloudWatch Stream, Firehose and an S3 Bucket.
Overall, if you and your business relies on AWS infrastructure, the value that can be extracted from the new ingestion architecture overweights the cons of having more pieces to setup.
As always, stay tuned for more updates on this project as I dig into the internals on the improvements that can be made in the metric conversion per-se.
The Unsatisfying State of Prometheus-Based Monitoring of AWS Infrastructure
Originally posted in LinkedIn
Introduction
Prometheus has become the de-facto industry standard for monitoring systems. It is a well-established open-source toolkit to collect, store and query metrics data and also trigger alerts based on them. Prometheus has a large support community and most open-source services expose their metrics using Prometheus compatible metrics or have tooling around to make them available in a Prometheus scrapeable format.
On the Cloud provider front, Amazon AWS has been - and still is - the leader in terms of market share[1]. A large number of businesses heavily rely not only in the infrastructure they provide, but in the hosted and proprietary services they sell (DynamoDB, SQS, etc). AWS itself provides a product for monitoring their solutions: AWS CloudWatch. Unsurprisingly, one can use CloudWatch to store custom metrics but CloudWatch is the only way of accessing metrics generated by AWS Services.
It is common to see scenarios where companies have a partitioned monitoring solution. The applications, services and self-hosted pieces of infrastructure are monitored using a Prometheus-centered solution (Prometheus, Grafana, AlertManager, etc) while the proprietary services they use are monitored through CloudWatch.
This is not ideal for several reasons. Primarily the two solutions are really different in a lot of dimensions, thus making it more difficult to have a workforce that is comfortable with both systems and is able to efficiently use either depending on the use case. Training is basically doubled as the concepts are not really transferable from one solution to the other. Also, having a partitioned monitored solution also makes it harder to correlate metrics that are not in the same system for obvious reasons. I think it is a fair assumption to think that companies will have a desire to try to centralize their monitoring solution into a one-stop shop as much as possible.
I would argue that centralizing into CloudWatch is not the common - or even the right - approach. The number of things without native Prometheus support is smaller than the number of things that don’t have any CloudWatch support. Also, if deciding to unify, I’d rather be unifying towards an industry standard that is more commonly used than a proprietary tool.
Current Tooling Available
If the decision has been made to unify the metrics on the Prometheus side, then there’s the need to import the CloudWatch metrics into Prometheus. The current two ways I have found to be available are either Prometheus’ CloudWatch Exporter or YACE. With both it is possible to transform metrics exposed by AWS CloudWatch into Prometheus scrape-able metrics. And even though they differ in some ways, they both share the same underlying flaws that make their output not too valuable.
The issues stem from the trade-offs taken by the developers of these tools and what they set out to achieve. I would summarize these tools as general frameworks that transform any AWS CloudWatch Metrics into a Prometheus scrapeable format. They don’t aim to understand the semantics of each AWS CloudWatch provides and what it is representing; this greatly simplifies the maintenance, but comes with limitations. Also, the decision to retrieve the metrics through the AWS API rather than other available mechanisms was likely because it makes the tool and its deployment model simpler.
The first and most important of these, is a conceptual flaw. They obtain an aggregated metric from AWS CloudWatch using their API and expose what they obtained as a gauge metric. All the metrics exposed through these exporters are gauges, independently of what they represent. For some of the metrics - such as number of ECS tasks running - this is OK but for most of them (lambda invocation count, ALB request count, etc) other Prometheus metric types would be more appropriate such as counters or summaries. Because of this design, rates cannot really be calculated and doing interpolation is not possible.
Secondly, the trade-offs of the configured scraping frequency, lookback period and the delay has major impacts in the retrieved metrics as well as the performance. Given that these exporters work by periodically polling the CloudWatch API and that AWS doesn’t make the metrics immediately available through the API, a mismatch in these settings can lead to different non-optimal situations. Setting the frequency and lookback periods too low can lead to metrics with tons of gaps as they will not be available at the frequency they are requested. Using the sum metric with a lookback period larger than scraping frequency will lead to “overlapping” datapoints; normalizing to rates can be done in PromQL but that will depend on the lookback period used. Setting the update frequency too high in a large infrastructure footprint can lead to the API calls being throttled and/or the exporter not being able to finish in time leading to metrics with gaps in them. But lowering the frequency, increasing the period and adding a delay can lead to an intolerable lag in the metric becoming available. For some metrics I have observed an average lag of 15 minutes to make it reliable.
Conclusion
Overall, the available tools I found that are capable to retrieve and expose CloudWatch metrics in a way that can be scraped by Prometheus is quite unfulfilling. There are these two exporters that are able to provide a basic level of translation which is definitely better than nothing. But for enterprises where monitoring is business-critical, the shortcomings of the features the provide are noticeable. The constraints placed by the way the metrics are exposed as well as the lag in the metric availability makes it harder to centralize monitoring solely in Prometheus and falling back to using CloudWatch directly seems to be result.